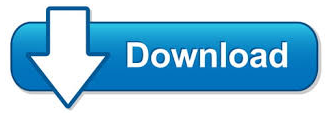
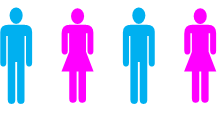
In turn, that the collected data often doesn't reflect reality, contains typos, or is stored in the wrong format.ĭata cleansing refers to the processes employed to validate and correct data. Often because the data scientist's requirements simply aren't known yet when the data is collected. Unfortunately - data quality is often not considered at the source. That is why data cleansing has become an increasingly important topic.

You're expecting it, so here it is: garbage in = garbage out. If the data isn't good, the insights won't be good either. A prerequisite for that magic - is that the data is good. They apply their data science magic to make this happen. A data scientist's goal is to leverage the data to create insights. Let alone, the requirements that the data scientist carrying out that analysis has for the data. When data is collected, the system or person collecting it often doesn't know that later on it will be used for analysis.
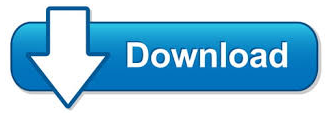